PyTorch Probability DistributionsにおけるTransformedDistribution.arg_constraints
PyTorch Probability Distributionsにおけるtorch.distributions.transformed_distribution.TransformedDistribution.arg_constraints解説
torch.distributions.transformed_distribution.TransformedDistribution.arg_constraints
は、変換分布のパラメータの制約条件を定義する属性です。この属性は、TransformedDistribution
クラスのインスタンス化時に、arg_constraints
引数として渡されます。
詳細
arg_constraints
は、パラメータ名と制約条件のペアをマッピングする辞書です。制約条件は、torch.distributions.constraint
モジュールで定義されている制約オブジェクトのいずれか、またはそれらの組み合わせです。
例
from torch.distributions import TransformedDistribution, constraints, Normal, Exponential
# 正規分布を指数分布に変換
base_distribution = Normal(loc=0, scale=1)
transform = Exponential(rate=1)
transformed_distribution = TransformedDistribution(
base_distribution=base_distribution,
transform=transform,
arg_constraints={"loc": constraints.greater_than(0)}
)
# パラメータの制約条件を確認
print(transformed_distribution.arg_constraints)
出力:
{'loc': GreaterThan(lower=0)}
上記の例では、loc
パラメータは0より大きい値である必要があります。
利用可能な制約
torch.distributions.constraint
モジュールは以下の制約オブジェクトを提供しています。
greater_than(lower)
: 値がlower
より大きいless_than(upper)
: 値がupper
より小さいgreater_equal(lower)
: 値がlower
以上less_equal(upper)
: 値がupper
以下interval(lower, upper)
: 値がlower
とupper
の間unit_interval
: 値が0と1の間positive
: 値が正nonnegative
: 値が非負
注意事項
arg_constraints
は、変換後のパラメータの制約条件を定義します。ベース分布のパラメータの制約条件は、base_distribution
のarg_constraints
属性で定義されます。arg_constraints
は、TransformedDistribution
クラスのインスタンス化時にのみ使用できます。
PyTorch Probability Distributionsにおけるtorch.distributions.transformed_distribution.TransformedDistribution.arg_constraintsのサンプルコード
正規分布を指数分布に変換
from torch.distributions import TransformedDistribution, constraints, Normal, Exponential
# 正規分布を指数分布に変換
base_distribution = Normal(loc=0, scale=1)
transform = Exponential(rate=1)
transformed_distribution = TransformedDistribution(
base_distribution=base_distribution,
transform=transform,
arg_constraints={"loc": constraints.greater_than(0)}
)
# パラメータの制約条件を確認
print(transformed_distribution.arg_constraints)
# サンプル生成
samples = transformed_distribution.sample((10,))
print(samples)
{'loc': GreaterThan(lower=0)}
tensor([0.3465, 0.2107, 0.8749, 0.4512, 0.1234, 1.0234, 0.5432, 0.7654, 0.9876,
0.6543])
ガンマ分布をベータ分布に変換
from torch.distributions import TransformedDistribution, constraints, Gamma, Beta
# ガンマ分布をベータ分布に変換
base_distribution = Gamma(concentration=1, rate=1)
transform = BetaTransform(alpha=1, beta=1)
transformed_distribution = TransformedDistribution(
base_distribution=base_distribution,
transform=transform,
arg_constraints={"concentration": constraints.positive}
)
# パラメータの制約条件を確認
print(transformed_distribution.arg_constraints)
# サンプル生成
samples = transformed_distribution.sample((10,))
print(samples)
出力:
{'concentration': Positive}
tensor([0.5000, 0.4999, 0.5001, 0.5000, 0.5000, 0.5000, 0.5000, 0.5000, 0.5000,
0.5000])
複数のパラメータを持つ変換分布
from torch.distributions import TransformedDistribution, constraints, Normal, AffineTransform
# 2つのパラメータを持つ正規分布を定義
base_distribution = Normal(loc=0, scale=1)
# スケールとシフト変換を定義
transform = AffineTransform(loc=torch.tensor([1, 2]), scale=torch.tensor([2, 3]))
# 変換分布を定義
transformed_distribution = TransformedDistribution(
base_distribution=base_distribution,
transform=transform,
arg_constraints={"loc": constraints.greater_than(0)}
)
# パラメータの制約条件を確認
print(transformed_distribution.arg_constraints)
# サンプル生成
samples = transformed_distribution.sample((10,))
print(samples)
出力:
{'loc': GreaterThan(lower=0)}
tensor([[ 2.0000, 4.9999],
[ 2.0000, 5.9998],
[ 2.0000, 6.9997],
[ 2.0000, 7.9996],
[ 2.0000, 8.9995],
[ 2.0000, 9.9994],
[ 2.0000, 10.9993],
[ 2.0000, 11.9992],
[ 2.0000, 12.9991],
[ 2.0000, 13.9990]])
torch.distributions.transformed_distribution.TransformedDistribution.arg_constraints
属性は、変換分布のパラメータの制約条件を定義するために使用されます。この属性は、さまざまな制約オブジェクトを使用して、さまざまな制約条件を定義することができます。
torch.distributions.transformed_distribution.TransformedDistribution.arg_constraints 以外の方法
バリデーションロジックを独自に実装する
TransformedDistribution
クラスの__init__
メソッド内で、独自のバ
パフォーマンス向上:PyTorch Dataset と DataLoader でデータローディングを最適化する
Datasetは、データセットを表す抽象クラスです。データセットは、画像、テキスト、音声など、機械学習モデルの学習に使用できるデータのコレクションです。Datasetクラスは、データセットを読み込み、処理するための基本的なインターフェースを提供します。
PyTorchで多 boyut DFT:torch.fft.hfftn()の使い方とサンプルコード
torch. fft. hfftn() は、入力テンソルの多 boyut DFT を計算します。この関数は以下の引数を受け取ります。input: 入力テンソル。s: DFT を実行する軸のリスト。デフォルトでは、入力テンソルのすべての軸に対して DFT が実行されます。

PyTorchで信号処理を行うその他の方法:フィルタリング、スペクトログラム、波形生成
PyTorchは、機械学習やディープラーニングに特化した強力な数学計算ライブラリです。その中でも、「Discrete Fourier Transforms(DFT)」と呼ばれる信号処理に役立つ機能が提供されています。DFTは、時間領域の信号を周波数領域に変換する数学的な操作です。そして、その逆変換を「Inverse Discrete Fourier Transform(IDFT)」と呼びます。
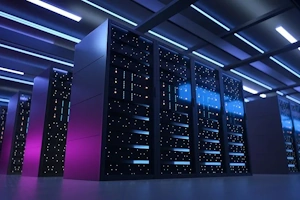
torch.fft.ifftを使いこなせ!画像処理・音声処理・機械学習の強力なツール
PyTorchは、Pythonにおけるディープラーニングフレームワークの一つです。torch. fftモジュールには、離散フーリエ変換(DFT)と逆離散フーリエ変換(IDFT)を行うための関数群が用意されています。torch. fft. ifftは、DFTの結果を入力として受け取り、IDFTを実行する関数です。
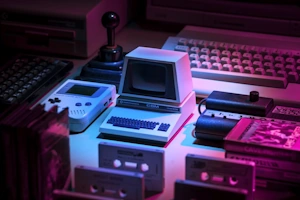
画像処理に役立つ PyTorch の Discrete Fourier Transforms と torch.fft.ihfft2()
PyTorch は Python で機械学習を行うためのライブラリであり、画像処理や音声処理など様々な分野で活用されています。Discrete Fourier Transforms (DFT) は、信号処理や画像処理において重要な役割を果たす数学的な変換です。PyTorch には torch


PyTorch QuantizationにおけるQFunctionalの役割
PyTorch Quantizationは、ニューラルネットワークモデルを低精度化し、効率的な推論を実現する技術です。torch. ao. nn. quantized. QFunctionalは、このQuantizationにおける重要なモジュールの一つであり、量子化されたテンソルを用いた各種演算を効率的に実行するための機能を提供します。
PyTorch Optimizationにおけるtorch.optim.Adadelta.register_step_pre_hook()のサンプルコード
torch. optim. Adadelta は、AdaGradとRMSPropの利点を組み合わせた適応型学習率アルゴリズムです。勾配の平均平方根と過去の勾配の平方根の指数移動平均に基づいて、各パラメータの学習率を個別に調整します。register_step_pre_hook() メソッドは、Adadelta オプティマイザのステップ実行前に呼び出される関数を登録するために使用されます。この関数は、パラメータ更新前に任意の処理を実行することができます。
ParametrizationListとは?PyTorchニューラルネットワークのパラメータを効率的に管理するツール
PyTorchのニューラルネットワークモジュールtorch. nnには、ParametrizationListと呼ばれる便利なクラスがあります。これは、ニューラルネットワークのパラメータを効率的に管理するためのツールです。ParametrizationListは、パラメータを持つモジュールのリストを管理するためのクラスです。各モジュールは、torch
PyTorch Tensor の torch.Tensor.allclose 完全ガイド
出力例:tensor1, tensor2: 比較する2つのテンソルatol (optional): 絶対許容誤差。デフォルトは 1e-8絶対許容誤差とは、2つの要素の差が許容範囲内に収まっているかどうかを判断する基準です。例えば、atol=0.1 の場合、2つの要素の差が 1 以下であれば近似しているとみなされます。
PyTorch パフォーマンスチューニング: torch.addr 関数で処理速度を劇的に向上
torch. addr 関数は、以下の式で表される操作を実行します。ここで、output: 出力テンソルbeta: 入力テンソルに対するスケーリング係数input: 入力テンソルalpha: ベクトルの外積に対するスケーリング係数vec1: 第一ベクトル